My blog posts are largely a mechanism for me to think aloud about retirement finance and to receive helpful feedback from my readers. I’m learning, not teaching, and I learned a lot in 2015.
I published 44 posts on this blog over the past year averaging about 958 words per post, or a little more than 42,000 words. I also co-authored a paper on portfolio ruin with my son and daughter, which I expect to publish early in 2016, that adds another 4,000 words (many of which I didn’t know before we wrote the paper), plus a few posts at Advisor Perspectives. I don’t even want to estimate the number of lines of R code and Mathematica code I wrote this year and there were a few presentations at conferences. Let's just ballpark it at 50,000 words.
(A classic novel is typically 80 to 100 thousand words; Fahrenheit 451 is a little over 46,000.)
That’s a lot of words for a hobby, a lot of lattes and a lot of learning.
My audience is diverse and knowledgeable and teaches me a lot, often by simply asking the right questions. Nearly 86% of them log on from the US, 4% log on in the UK and 1.8% from Canada. The surprise, however, is that 3% of my readers log on from Ukraine and nearly 2% from Russia. I extend a heartfelt Дякую! to the former and Спасибо! to the latter.
(I really hope I got that right. A Russian-speaking friend confirmed one, but my only Ukrainian friend moved to South Carolina last summer.)
Here are a few of the important things I learned in 2015.
There is no dispute between Jeremy Siegel and Zvi Bodie about stocks becoming safer the longer you hold them. They don’t.
I also learned that people have a difficult time giving up beliefs about finance. I still have planners argue that stocks become safer. Spending only dividends is not a valuable retirement income strategy and risk of ruin is primarily useful only as a research tool. A bond ladder held to maturity and a bond fund are not the same thing. A ladder of TIPS bonds held to maturity is essentially cash.
My son’s potato casserole is outstanding, but should be baked in a disposable dish. (I wash the dishes at our house.)
I learned that time segmentation (“bucket”) strategies can’t be depended upon to avoid a bad sequence of returns. They might, but it isn’t a sure thing.
I received an award for a paper in Indianapolis this summer. I had not visited the city for decades and I learned that it is still incredibly flat. I also learned that a small replica of Rodin’s “The Thinker” in your carry-on looks like a bunny rabbit on the x-ray screen of a TSA employee. ("Are you sure it's not a bunny rabbit? It really looks like a bunny rabbit.")
Game theory can be a useful way to think about retirement strategies.
I learned that even decaffeinated coffee after 3 pm can impact my evening’s sleep. My wife insisted that I test this theory and, much to my chagrin, she was proven correct. This dramatically altered my afternoon writing strategy at Caffe Driade.
Retirement income systems may be chaotic and virtually impossible to predict except in equilibrium, so chaos theory is another useful way to view retirement finance.
I learned that co-authoring a research paper with a son you taught to play basketball and who taught you how to play Super Mario World, and a daughter you taught to fish and who danced with you at her wedding is one of the coolest things that you will ever do.
Even with a basic assumption that our portfolio will return 5% with a standard deviation of 12%, the range of reasonable likely outcomes is too broad to effectively choose among spending rates and asset allocations.
Retirement income models probably involve a lot more uncertainty than most people assume.
I learned that academic papers on retirement finance are often misinterpreted, not just by retirees, but by financial planners, as well. Examples include "retirement spending looks like a smile" (the rate of annual spending change looks like a smile, but actual spending typically declines throughout retirement) and “retirees should increase their asset allocation as they age” (it depends – a custom asset allocation plan is always preferable).
I took the GMAT 30 years ago to get my MBA and again this past year. Perhaps most fun of all, I learned in 2015 that I can still outperform 4 out of 5 young whippersnappers taking the exam.
Old guys rule.
Here’s to an educational 2016.
In my next post, I'll explain Why Retirees Go Broke.
Monday, December 28, 2015
Friday, December 18, 2015
Retirement Income and Chaos Theory
Are retirement spending models chaotic? In my last post, Positive Feedback Loops: The Other Roads to Ruin, I pointed out the exposure to the risk of these loops in at least three aspects of typical retirement income models: market returns, spending from a volatile portfolio, and the total and rapid collapse of a large real estate portfolio. I noticed these loops because I have an amateur interest in chaos theory, and as I mentioned at the end of that post, positive feedback loops are characteristic of chaotic systems.
Whether or not retirement income systems are chaotic is an important issue because chaotic systems are riskier than stochastic (probabilistic) systems. We tend to study retirement income systems with probabilities. If the systems are chaotic, they're riskier than inferential statistics (probabilities) suggests. Bear with me through some background and I will explain the relevance to your retirement plan.
According to the website, FractalFoundation.org, “chaos is the science of surprises, of the nonlinear and the unpredictable. It teaches us to expect the unexpected.” Most retirement income research uses the science of probabilities and statistics that reveal what is unlikely, but not necessarily what is unexpected.
As early as 1887, Henri Poincaré showed that while Newtonian physics could perfectly predict the orbit of two planetary bodies, adding a third body to the mix turned a straightforward problem into one that is virtually unsolvable. A system as simple as the double pendulum simulated below can exhibit chaotic behavior. Its trajectory varies dramatically with small changes in its initial position. Probabilities won't predict the trajectory of the double pendulum because we can't know precisely enough where it will start. As the three body problem and the double pendulum show, systems don't have to be complex to behave chaotically.
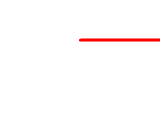
When I study the models of retirement income studies, I see a number of characteristics of the models that are also characteristics of chaotic, not probabilistic, systems.
Let’s look at those characteristics as suggested by FractalFoundation.org.
Unpredictability. “Because we can never know all the initial conditions of a complex system in sufficient detail, we cannot hope to predict the ultimate fate of a complex system.” Like the starting point of the double pendulum, we can’t predict precisely enough where we are in the cycle of future market returns at the outset of retirement. Is the market overvalued? Undervalued? Did we pick a fortuitous retirement date for the sequence of future returns? Only time will tell.
The Transition between Order and Disorder. “Chaos is not simply disorder. Chaos explores the transitions between order and disorder, which often occur in surprising ways.” Chaotic systems, such as the stock market may be, can remain in a stable state for long periods of time before inexplicably becoming unstable. The 2010 Flash Crash is an example that is still not well-explained. In the example I provided in my previous post, two households went from well-to-do to bankrupt in less than a year when the U.S. economy crashed in late 2007. Everything looked fine for both families just months earlier.
Mixing. “Turbulence ensures that two adjacent points in a complex system will eventually end up in very different positions after some time has elapsed. Two neighboring water molecules may end up in different parts of the ocean or even in different oceans." Look at the range of outcomes for a retirement portfolio balance simulation in the chart below. One scenario depleted the portfolio in just 19 years while another grew to more than $6M. Each scenario started at the same point (a $1M portfolio balance) under the same initial conditions.
Positive Feedback Loops. “Systems often become chaotic when there is feedback present. A good example is the behavior of the stock market. As the value of a stock rises or falls, people are inclined to buy or sell that stock. This in turn further affects the price of the stock, causing it to rise or fall chaotically.” See my previous post on positive feedback loops for retirement examples.
Here are more characteristics of chaotic systems not included in the Fractal Foundation's list.
Attractors. An attractor is a state toward which a system tends to evolve from a wide variety of starting conditions. System values that get close enough to the attractor tend to remain close to it. A type called a "fixed point attractor", which attracts trajectories to a single point, describes portfolio ruin.
Here's a graphical depiction of a point attractor from Young Scientists Journal. Imagine this as two portfolio balance trajectories that enter a positive feedback loop and spiral downward to ruin.
(Want to see something really cool? Google "images of strange attractors" and you will find some amazing graphics, like this.)
Prediction Horizons. Another characteristic of chaotic systems is a prediction horizon, explained by Professor Jonathan Borwein.
As the Terminal Wealth chart above shows, the prediction horizon for retirement portfolio balances is a less than a year, beyond which the outcomes diverge dramatically and become much more uncertain.
Experts disagree on an exact definition of chaotic systems. They tend to describe their characteristics, instead, much in the way Supreme Court Justice, Potter Stewart once described obscenity – “I know it when I see it.” I'm not an expert in chaos theory, but when I consider the characteristics in common with retirement income models, I think I see it.
My interest in chaos theory is limited to popular books on the subject because the math, differential equations and fractal geometry, is pretty demanding. So, I posed several questions to chaos theory expert, Tom Konrad, who has a doctorate in complex analysis and chaos theory and edits AltEnergyStocks.com. I described spending from a volatile portfolio to Dr. Konrad and asked if he thought it might be a chaotic system.
“It's impossible to ‘prove' that a system is chaotic or is not when we don't completely understand the underlying mechanisms,” he explained.
“It certainly displays chaotic characteristics”, he continued, “but other than acknowledging that, I'm not sure if anything would be accomplished by quantifying them.”
In my interpretation, if it quacks like a duck and tastes like a duck, dinner probably won’t suffer if mathematicians can’t agree to the precise extent of its duck-ness. If the retirement income system displays chaotic characteristics, there may be limited practical negative consequences to treating it as chaotic and it is safer to assume that it is.
Now, why is it important to understand if retirement income systems are chaotic or simply probabilistic? Because stochastic systems are unpredictable but statistically quantifiable, while complex and chaotic systems are even more unpredictable. It was on this point that Dr. Konrad provided my favorite explanation.
Retirement income studies tend to use probabilities to focus on long-term sustainability of savings as a function of market volatility alone. This approach won't catch many quickly developing expense-related crises, especially since the studies tend to ignore expense uncertainty altogether. When we say a retiree has a 5% risk of outliving her savings, we mean a 5% risk of outliving savings due solely to market volatility. But, there are other risks to those savings that should also be considered.
These studies explain long, slow declines in standard of living, not catastrophic failures, in a world where market returns are normally distributed and mean-reverting and no one ever needs to spend more than their "sustainable withdrawal." Their recommendations – diversification and spending adjustments – provide little help in a spending crisis.
Chaos theory helps explain household finances that veer suddenly from normal equilibrium into a crisis. Debt, divorce or some other expense shock shoves the portfolio balance trajectory into a positive feedback loop and toward the point attractor that is portfolio ruin.
Take another look at the green trajectories in the spiral above and consider the households from my previous post that went from equilibrium to bankruptcy and, in one case divorce, in less than a year. This is not the stuff of 30-year Monte Carlo simulations of normally distributed market returns.
Probabilities and equilibrium are important parts of the story, but they aren't the entire story.
I admit this post is a bit dense, particularly if you have no interest in chaos theory. But, if you take away the following, I think you'll be fine. When a planner tells you that you have a 5% probability of depleting your savings, she typically means a 5% probability of going broke as a result of market volatility. Alas, there are other ways to go broke. If spending systems are chaotic, which I suspect but can't prove mathematically, there are conditions under which their outcomes are unpredictable and probabilities don't help. And lastly, as Dr. Konrad suggests, if they behave chaotically, we might not gain much by proving how chaotic they are.
Unless and until we know that these systems are not chaotic, the safest path for a retiree would be to assume that they are and that the probability of ruin is greater than studies have indicated.
Once again, I note that my posts about market risk shouldn't be taken as an argument against investing in stocks. Retirement income without equities is terribly expensive. But it's important to understand the risks and to be prepared to deal with them. There's more to worry about than a bad sequence of returns and living too long.
Next time, I'll sum up what I learned about retirement finance in 2015.
Looking for some good popular books on chaos theory without the differential equations? Try The Black Swan, Fooled by Randomness, Chaos: Making a New Science, or Dr. Konrad's column in Forbes.
Whether or not retirement income systems are chaotic is an important issue because chaotic systems are riskier than stochastic (probabilistic) systems. We tend to study retirement income systems with probabilities. If the systems are chaotic, they're riskier than inferential statistics (probabilities) suggests. Bear with me through some background and I will explain the relevance to your retirement plan.
According to the website, FractalFoundation.org, “chaos is the science of surprises, of the nonlinear and the unpredictable. It teaches us to expect the unexpected.” Most retirement income research uses the science of probabilities and statistics that reveal what is unlikely, but not necessarily what is unexpected.
[Tweet this]Are retirement spending models chaotic?
As early as 1887, Henri Poincaré showed that while Newtonian physics could perfectly predict the orbit of two planetary bodies, adding a third body to the mix turned a straightforward problem into one that is virtually unsolvable. A system as simple as the double pendulum simulated below can exhibit chaotic behavior. Its trajectory varies dramatically with small changes in its initial position. Probabilities won't predict the trajectory of the double pendulum because we can't know precisely enough where it will start. As the three body problem and the double pendulum show, systems don't have to be complex to behave chaotically.
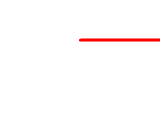
When I study the models of retirement income studies, I see a number of characteristics of the models that are also characteristics of chaotic, not probabilistic, systems.
Let’s look at those characteristics as suggested by FractalFoundation.org.
Unpredictability. “Because we can never know all the initial conditions of a complex system in sufficient detail, we cannot hope to predict the ultimate fate of a complex system.” Like the starting point of the double pendulum, we can’t predict precisely enough where we are in the cycle of future market returns at the outset of retirement. Is the market overvalued? Undervalued? Did we pick a fortuitous retirement date for the sequence of future returns? Only time will tell.
The Transition between Order and Disorder. “Chaos is not simply disorder. Chaos explores the transitions between order and disorder, which often occur in surprising ways.” Chaotic systems, such as the stock market may be, can remain in a stable state for long periods of time before inexplicably becoming unstable. The 2010 Flash Crash is an example that is still not well-explained. In the example I provided in my previous post, two households went from well-to-do to bankrupt in less than a year when the U.S. economy crashed in late 2007. Everything looked fine for both families just months earlier.
Mixing. “Turbulence ensures that two adjacent points in a complex system will eventually end up in very different positions after some time has elapsed. Two neighboring water molecules may end up in different parts of the ocean or even in different oceans." Look at the range of outcomes for a retirement portfolio balance simulation in the chart below. One scenario depleted the portfolio in just 19 years while another grew to more than $6M. Each scenario started at the same point (a $1M portfolio balance) under the same initial conditions.
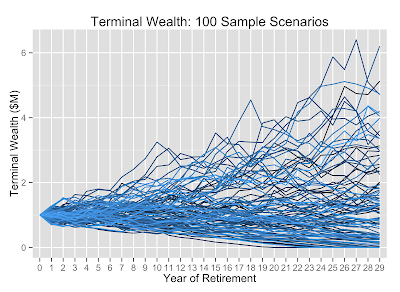
Positive Feedback Loops. “Systems often become chaotic when there is feedback present. A good example is the behavior of the stock market. As the value of a stock rises or falls, people are inclined to buy or sell that stock. This in turn further affects the price of the stock, causing it to rise or fall chaotically.” See my previous post on positive feedback loops for retirement examples.
Here are more characteristics of chaotic systems not included in the Fractal Foundation's list.
Attractors. An attractor is a state toward which a system tends to evolve from a wide variety of starting conditions. System values that get close enough to the attractor tend to remain close to it. A type called a "fixed point attractor", which attracts trajectories to a single point, describes portfolio ruin.
Here's a graphical depiction of a point attractor from Young Scientists Journal. Imagine this as two portfolio balance trajectories that enter a positive feedback loop and spiral downward to ruin.
(Want to see something really cool? Google "images of strange attractors" and you will find some amazing graphics, like this.)
Prediction Horizons. Another characteristic of chaotic systems is a prediction horizon, explained by Professor Jonathan Borwein.
“What at first glance appears to be random behavior is completely deterministic – it only seems random because imperceptible changes are making all the difference. The rate at which these tiny differences stack up provides each chaotic system with a prediction horizon – a length of time beyond which we can no longer accurately forecast its behavior. In the case of the weather, the prediction horizon is nowadays about one week.”
Experts disagree on an exact definition of chaotic systems. They tend to describe their characteristics, instead, much in the way Supreme Court Justice, Potter Stewart once described obscenity – “I know it when I see it.” I'm not an expert in chaos theory, but when I consider the characteristics in common with retirement income models, I think I see it.
My interest in chaos theory is limited to popular books on the subject because the math, differential equations and fractal geometry, is pretty demanding. So, I posed several questions to chaos theory expert, Tom Konrad, who has a doctorate in complex analysis and chaos theory and edits AltEnergyStocks.com. I described spending from a volatile portfolio to Dr. Konrad and asked if he thought it might be a chaotic system.
“It's impossible to ‘prove' that a system is chaotic or is not when we don't completely understand the underlying mechanisms,” he explained.
“It certainly displays chaotic characteristics”, he continued, “but other than acknowledging that, I'm not sure if anything would be accomplished by quantifying them.”
In my interpretation, if it quacks like a duck and tastes like a duck, dinner probably won’t suffer if mathematicians can’t agree to the precise extent of its duck-ness. If the retirement income system displays chaotic characteristics, there may be limited practical negative consequences to treating it as chaotic and it is safer to assume that it is.
Now, why is it important to understand if retirement income systems are chaotic or simply probabilistic? Because stochastic systems are unpredictable but statistically quantifiable, while complex and chaotic systems are even more unpredictable. It was on this point that Dr. Konrad provided my favorite explanation.
“Chaotic systems are less predictable than stochastic systems. Sufficient historical data will eventually allow you to quantify a stochastic system; this is not true for a chaotic system. The stock market seems to be un-quantifiable based on the historic record. That does not necessarily mean that it is chaotic (although there are other reasons, such as positive feedback loops, to believe that it is) but it is clearly harder to quantify than a stochastic system would be.”We debate whether 200 years of stock market returns are enough to characterize the returns of the market's internal processes, or its impact on retirement plans. If the system is chaotic, we will never have enough historical data to make it predictable.
Retirement income studies tend to use probabilities to focus on long-term sustainability of savings as a function of market volatility alone. This approach won't catch many quickly developing expense-related crises, especially since the studies tend to ignore expense uncertainty altogether. When we say a retiree has a 5% risk of outliving her savings, we mean a 5% risk of outliving savings due solely to market volatility. But, there are other risks to those savings that should also be considered.
These studies explain long, slow declines in standard of living, not catastrophic failures, in a world where market returns are normally distributed and mean-reverting and no one ever needs to spend more than their "sustainable withdrawal." Their recommendations – diversification and spending adjustments – provide little help in a spending crisis.
Chaos theory helps explain household finances that veer suddenly from normal equilibrium into a crisis. Debt, divorce or some other expense shock shoves the portfolio balance trajectory into a positive feedback loop and toward the point attractor that is portfolio ruin.
Take another look at the green trajectories in the spiral above and consider the households from my previous post that went from equilibrium to bankruptcy and, in one case divorce, in less than a year. This is not the stuff of 30-year Monte Carlo simulations of normally distributed market returns.
Probabilities and equilibrium are important parts of the story, but they aren't the entire story.
I admit this post is a bit dense, particularly if you have no interest in chaos theory. But, if you take away the following, I think you'll be fine. When a planner tells you that you have a 5% probability of depleting your savings, she typically means a 5% probability of going broke as a result of market volatility. Alas, there are other ways to go broke. If spending systems are chaotic, which I suspect but can't prove mathematically, there are conditions under which their outcomes are unpredictable and probabilities don't help. And lastly, as Dr. Konrad suggests, if they behave chaotically, we might not gain much by proving how chaotic they are.
Unless and until we know that these systems are not chaotic, the safest path for a retiree would be to assume that they are and that the probability of ruin is greater than studies have indicated.
Once again, I note that my posts about market risk shouldn't be taken as an argument against investing in stocks. Retirement income without equities is terribly expensive. But it's important to understand the risks and to be prepared to deal with them. There's more to worry about than a bad sequence of returns and living too long.
Next time, I'll sum up what I learned about retirement finance in 2015.
Looking for some good popular books on chaos theory without the differential equations? Try The Black Swan, Fooled by Randomness, Chaos: Making a New Science, or Dr. Konrad's column in Forbes.
Friday, December 11, 2015
Positive Feedback Loops: The Other Roads to Ruin
Positive feedback like, “Nice post!” is always welcome, but positive
feedback that gets stuck in a loop can have catastrophic results.
The most memorable experience of positive feedback is the head-splitting screech that fills an auditorium when the PA system experiences feedback. Someone speaks into the microphone and the sound is amplified. Amplified sound from the speakers is picked up by the microphone and amplified again through the speakers and back to the microphone and . . . well, by about the fifth cycle through the amp everyone in the room is holding their hands over their ears and mouthing silent profanities.
The most common experience of a negative feedback loop is your home thermostat that reduces heat when the temperature gets higher. You’d think that a screeching PA system would be negative and a thermostat controlling your home heating and cooling would be positive, but that isn’t how feedback loops are named.
According to one website, a more formal explanation of the difference is this:
To answer that, I'll tell a story about a retired couple in 2007, because I was raised in the South where every question is answered with a story. My story is not strictly true (a long-standing tradition of Southern stories). I will build a composite retired household from personal observations of multiple actual households from that time, some of whom weren't really even retired but, as my grandfather would’ve said with a grin if he were telling this story, “they might'a been.”
Jim and Linda retired in Omaha in 2005. The family seemed well-to-do, but they lived in a large, heavily mortgaged home. Jim had built a small fortune over his working career investing in rental homes, also heavily mortgaged, and most of their retirement income came from those properties. They both had retirement accounts invested in index funds worth about $200,000 and significant income from several CD’s and a money market fund.
Life went pretty much as planned until late 2007 when the stock market began a 50%, 18-month drop, interest paid on safe investments dropped to zero and, worse for Jim and Linda, the real estate market tanked, all simultaneously. Though most people would eventually recover from these crises, Jim and Linda wouldn't – a financial crisis can be a very personal thing.
With the rental income gone and the CD's and money market funds now paying a pitiful fraction of one percent, Jim could no longer afford the mortgage on his home plus the mortgages on fifty or so rental properties so he let the unprofitable rentals go. There were no buyers, so “letting go” meant skipping mortgage payments until the banks foreclosed.
The family’s rental business had drifted into a positive feedback loop wherein foreclosed properties reduced income, which reduced their ability to pay other mortgages, which resulted in more foreclosures, which resulted in even less income. It didn't stop until all the properties, including their home, were foreclosed. In less than a year, Jim and Linda's once-shiny finances looked like PA system feedback sounds.
The couple’s plan had assumed that their portfolio of rental properties was well diversified. Sure, a few of the fifty properties might fail from time to time, but what were the odds that a catastrophic number of them would fail in short order? Incidentally, this is the same assumption that led to the Wall Street collateralized mortgage obligations crisis on a much grander scale and as Jim, Linda and several Wall Street giants soon learned, the correlations had been much higher than they had believed.
Jim needed to sell their stocks, intended to fund decades of retirement, at lower and lower prices as the stock market continued its collapse. The stock market would recover years later, as stock brokers promise it always will, but Jim and Linda wouldn't participate in that recovery. Their stocks had long been sold. The stock market had also entered a positive feedback loop with selling encouraging more selling for a year and a half.
Their retirement planner had told them that they could safely spend 4% of their portfolio value each year, or about $8,000. There was a small chance, around 5%, that a series of poor returns early in retirement would result in their outliving their savings, but an even smaller chance if they spent less when their portfolio declined in value. Unfortunately, they did realize that unlikely poor performance right after they retired.
Jim, Linda and their planner had assumed that spending might need to be reduced during a bad market spell. (We say “spell” in this context a lot in Southern stories. It’s a very versatile word. We can sit for a spell, Uncle Howard can have one of his spells, or in Louisiana we might even cast one.) What they hadn’t considered is that forces other than market volatility – like expense surprises and lost income, for example – might preclude their ability to make those needed spending cuts.
Each year that Jim and Linda spent more than planned from their portfolio increased their probability of ruin. The continual shrinking of their portfolio value as the market fell increased it even more. Soon that probability of ruin was a lot more than the original 5%. An example is provided in the table below.
Their portfolio spending strategy had drifted into a positive feedback loop (that's three if you're keeping score), wherein every year that they overspent from their savings they increased the risk of portfolio ruin, reduced their capacity to recover when the market did, and decreased the amount of spending that would be considered safe the following year, increasing the probability that they would need to overspend yet again. The way to escape this loop would have been to reduce spending from their portfolio. Without the rental income and fixed income interest, they couldn’t.
The good (and coincidentally true) ending to this story is that today, eight years later, all of the households in this composite have recovered to some degree, though one middle-aged couple divorced under the strain. The investment portfolio is gone, as is the real estate wealth, but they have a "spell" to recover because, as I said, none of them were actually retired. The outcome would have been worse had they been. Younger people have more risk capacity and remaining human capital.
Most people survived and completely recovered from these simultaneous stock, interest rate and real estate crashes, but Jim and Linda did not. Had their investment properties not simultaneously tanked, they would have easily survived the bear market and kept their home.
What types of spending shocks can initiate a positive feedback loop? There are several candidates. A late-life divorce can be financially devastating and, though you may imagine them rare, they are not. I am aware of three among my circle of acquaintances over the past five years. (One gentleman was 80 and he married again within a year.)
Unexpected medical expenses, particularly onerous with dementia (see Costs for Dementia Care Far Exceeding Other Diseases), aren't uncommon. Two families within my circle of acquaintances are retired and financially stressed by the high support costs of their grown children who have medical problems. A combination of small underestimates of spending, expected market returns, and longevity could also do the trick in an otherwise survivable economic downturn. (As I showed in 100% Certain That We're Not Sure, there is no certainty that you picked the correct portfolio spending rate to begin with. You may already be overspending.)
Research shows that well-diversified retirement portfolios are unlikely to completely fail as a result of market volatility. (See, for example, Larry Frank.) The portion of the portfolio that is unlikely to disappear is sometimes referred to as a “soft floor.” Stout and Mitchell (download PDF) showed that retirees who reduce spending when their portfolio is stressed can reduce the risk of ruin by 30% to 40%.
The most memorable experience of positive feedback is the head-splitting screech that fills an auditorium when the PA system experiences feedback. Someone speaks into the microphone and the sound is amplified. Amplified sound from the speakers is picked up by the microphone and amplified again through the speakers and back to the microphone and . . . well, by about the fifth cycle through the amp everyone in the room is holding their hands over their ears and mouthing silent profanities.
The most common experience of a negative feedback loop is your home thermostat that reduces heat when the temperature gets higher. You’d think that a screeching PA system would be negative and a thermostat controlling your home heating and cooling would be positive, but that isn’t how feedback loops are named.
According to one website, a more formal explanation of the difference is this:
“Positive feedback loops enhance or amplify changes; this tends to move a system away from its equilibrium state and make it more unstable. Negative feedback loops tend to dampen or buffer changes; this tends to hold a system to some equilibrium state, making it more stable."So, when does a retirement income system “tend to move away from its equilibrium state and become unstable”?
To answer that, I'll tell a story about a retired couple in 2007, because I was raised in the South where every question is answered with a story. My story is not strictly true (a long-standing tradition of Southern stories). I will build a composite retired household from personal observations of multiple actual households from that time, some of whom weren't really even retired but, as my grandfather would’ve said with a grin if he were telling this story, “they might'a been.”
Jim and Linda retired in Omaha in 2005. The family seemed well-to-do, but they lived in a large, heavily mortgaged home. Jim had built a small fortune over his working career investing in rental homes, also heavily mortgaged, and most of their retirement income came from those properties. They both had retirement accounts invested in index funds worth about $200,000 and significant income from several CD’s and a money market fund.
Life went pretty much as planned until late 2007 when the stock market began a 50%, 18-month drop, interest paid on safe investments dropped to zero and, worse for Jim and Linda, the real estate market tanked, all simultaneously. Though most people would eventually recover from these crises, Jim and Linda wouldn't – a financial crisis can be a very personal thing.
[Tweet this]Market losses aren't the only road to ruin in retirement.
With the rental income gone and the CD's and money market funds now paying a pitiful fraction of one percent, Jim could no longer afford the mortgage on his home plus the mortgages on fifty or so rental properties so he let the unprofitable rentals go. There were no buyers, so “letting go” meant skipping mortgage payments until the banks foreclosed.
The family’s rental business had drifted into a positive feedback loop wherein foreclosed properties reduced income, which reduced their ability to pay other mortgages, which resulted in more foreclosures, which resulted in even less income. It didn't stop until all the properties, including their home, were foreclosed. In less than a year, Jim and Linda's once-shiny finances looked like PA system feedback sounds.
The couple’s plan had assumed that their portfolio of rental properties was well diversified. Sure, a few of the fifty properties might fail from time to time, but what were the odds that a catastrophic number of them would fail in short order? Incidentally, this is the same assumption that led to the Wall Street collateralized mortgage obligations crisis on a much grander scale and as Jim, Linda and several Wall Street giants soon learned, the correlations had been much higher than they had believed.
Jim needed to sell their stocks, intended to fund decades of retirement, at lower and lower prices as the stock market continued its collapse. The stock market would recover years later, as stock brokers promise it always will, but Jim and Linda wouldn't participate in that recovery. Their stocks had long been sold. The stock market had also entered a positive feedback loop with selling encouraging more selling for a year and a half.
Their retirement planner had told them that they could safely spend 4% of their portfolio value each year, or about $8,000. There was a small chance, around 5%, that a series of poor returns early in retirement would result in their outliving their savings, but an even smaller chance if they spent less when their portfolio declined in value. Unfortunately, they did realize that unlikely poor performance right after they retired.
Jim, Linda and their planner had assumed that spending might need to be reduced during a bad market spell. (We say “spell” in this context a lot in Southern stories. It’s a very versatile word. We can sit for a spell, Uncle Howard can have one of his spells, or in Louisiana we might even cast one.) What they hadn’t considered is that forces other than market volatility – like expense surprises and lost income, for example – might preclude their ability to make those needed spending cuts.
Each year that Jim and Linda spent more than planned from their portfolio increased their probability of ruin. The continual shrinking of their portfolio value as the market fell increased it even more. Soon that probability of ruin was a lot more than the original 5%. An example is provided in the table below.
Their portfolio spending strategy had drifted into a positive feedback loop (that's three if you're keeping score), wherein every year that they overspent from their savings they increased the risk of portfolio ruin, reduced their capacity to recover when the market did, and decreased the amount of spending that would be considered safe the following year, increasing the probability that they would need to overspend yet again. The way to escape this loop would have been to reduce spending from their portfolio. Without the rental income and fixed income interest, they couldn’t.
The good (and coincidentally true) ending to this story is that today, eight years later, all of the households in this composite have recovered to some degree, though one middle-aged couple divorced under the strain. The investment portfolio is gone, as is the real estate wealth, but they have a "spell" to recover because, as I said, none of them were actually retired. The outcome would have been worse had they been. Younger people have more risk capacity and remaining human capital.
Most people survived and completely recovered from these simultaneous stock, interest rate and real estate crashes, but Jim and Linda did not. Had their investment properties not simultaneously tanked, they would have easily survived the bear market and kept their home.
What types of spending shocks can initiate a positive feedback loop? There are several candidates. A late-life divorce can be financially devastating and, though you may imagine them rare, they are not. I am aware of three among my circle of acquaintances over the past five years. (One gentleman was 80 and he married again within a year.)
Unexpected medical expenses, particularly onerous with dementia (see Costs for Dementia Care Far Exceeding Other Diseases), aren't uncommon. Two families within my circle of acquaintances are retired and financially stressed by the high support costs of their grown children who have medical problems. A combination of small underestimates of spending, expected market returns, and longevity could also do the trick in an otherwise survivable economic downturn. (As I showed in 100% Certain That We're Not Sure, there is no certainty that you picked the correct portfolio spending rate to begin with. You may already be overspending.)
Research shows that well-diversified retirement portfolios are unlikely to completely fail as a result of market volatility. (See, for example, Larry Frank.) The portion of the portfolio that is unlikely to disappear is sometimes referred to as a “soft floor.” Stout and Mitchell (download PDF) showed that retirees who reduce spending when their portfolio is stressed can reduce the risk of ruin by 30% to 40%.
These studies don't
address spending volatility, however, so they don't predict what happens
if retirees, like Jim and Linda, are unable to make the needed spending reductions. Mean reversion
and diversification won't save those who can't due to divorce, dementia,
debt or dependents.
The positive feedback loops are present in these studies if you look for them carefully. Observe what happens to a portfolio in a constant-dollar spending model and you will see that when a portfolio declines in value and the retiree keeps spending a constant-dollar amount, she increases the risk of portfolio ruin going forward. When this happens several years in a row, the probability of ruin grows quite quickly. In the following table, a 65-year old couple in 1974 with a 50% equity portfolio wishing to spend $52,000 from a $1M portfolio each year would see their probability of ruin nearly triple in five years. (Probability of ruin calculated using Milevsky formula.)
(Another way to look at risk capacity is your portfolio spending rate. If you only need to spend 1% to 2% annually, you're far safer from spending crises than if you need to spend 4% or more annually.)
Most Southern stories have a moral and this one is no exception. Market volatility isn't the only road to portfolio depletion. Studies that ignore spending risk understate the probability that a retiree will outlive savings.
Here's my intuition: retirees are more likely to outlive their savings as the result of a spending crisis that triggers a downward, self-feeding spiral than they are to outlive their savings as a result of slowly overspending 4% a year instead of 3.5%, especially if they dynamically update their plans. I readily admit I don't have data to back that up, so take it for what it is. I also suspect that long-term persistent overspending and sequence risk are far more likely to result in a permanent loss of standard of living than outright ruin.
Year | Return on 50% Equity Portfolio | Portfolio Balance | Withdrawal Rate for $52k Spending |
Probability of Ruin |
---|---|---|---|---|
Initial | 1,000,000 | 5.0% | ||
1974 | -8% | 872,160 | 6.0% | 5.2% |
1975 | -11% | 729,942 | 7.1% | 8.5% |
1976 | 18.5% | 803,362 | 6.5% | 14.4% |
1977 | 6.5% | 800,200 | 6.5% | 10.6% |
1978 | -4% | 718,272 | 7.2% | 14.4% |
The table above demonstrates a positive feedback loop that would have developed had the retiree felt it was safe to continue the same amount of spending yearly as the market declined (it isn't), but it would also have developed if the retiree hadn't been able to reduce spending.
Positive feedback loops disappear in dynamic-updating models that assume that the retiree will be able to cut spending when necessary. Since there are no unmet liabilities (overspending) in these models, there are no positive feedback loops. The difference between probabilities of ruin between the two types of models offers an estimate of the risk of not being able to reduce spending.
Positive feedback loops disappear in dynamic-updating models that assume that the retiree will be able to cut spending when necessary. Since there are no unmet liabilities (overspending) in these models, there are no positive feedback loops. The difference between probabilities of ruin between the two types of models offers an estimate of the risk of not being able to reduce spending.
Stout and Mitchell found that adjusting spending reduces risk of ruin 30% to 40% compared to constant-dollar spending, so we can infer that not adjusting spending is about 43% to 67% riskier than adjusting it. The risk that we will deplete our portfolio because we can't adjust our spending is that 43% to 67% probability times the probability that we won't be able to reduce spending. The probability that we can't is far more difficult to estimate.
We can, however, ballpark that probability by considering our financial risk capacity. If we have lots of money saved relative to our spending needs, we have lots of risk capacity and the odds are better that we won't find ourselves unable to reduce spending from our portfolio when needed. The less savings we have relative to our spending, the greater the likelihood that we may be unable to reduce spending when necessary and the greater the chances that we will trigger a positive feedback loop that ends in ruin.
(Another way to look at risk capacity is your portfolio spending rate. If you only need to spend 1% to 2% annually, you're far safer from spending crises than if you need to spend 4% or more annually.)
Most Southern stories have a moral and this one is no exception. Market volatility isn't the only road to portfolio depletion. Studies that ignore spending risk understate the probability that a retiree will outlive savings.
Here's my intuition: retirees are more likely to outlive their savings as the result of a spending crisis that triggers a downward, self-feeding spiral than they are to outlive their savings as a result of slowly overspending 4% a year instead of 3.5%, especially if they dynamically update their plans. I readily admit I don't have data to back that up, so take it for what it is. I also suspect that long-term persistent overspending and sequence risk are far more likely to result in a permanent loss of standard of living than outright ruin.
Retirement income systems are vulnerable to positive feedback loops that can preclude the option to reduce spending in times of portfolio stress. Nor
is retirement uniquely the domain of positive feedback loops. My
personal experience with pre-retirement households that ended in
personal bankruptcy displayed evidence of loops. Long bouts of
unemployment or disability trigger them; consumer debt, divorce and
foreclosure combine to finish them, in good stock markets and bad.
Another impression I have is that most of us have far more confidence in retirement models than our understanding of them merits, and "us" includes financial planners. I appreciate models as research and learning tools and as tools that can support a good retirement plan, but I've been building and studying them for perhaps 20 years and the list of their weaknesses is long. They can't predict an individual household's future and they are not by themselves a retirement plan (see Michael Kitces' recent post.)
Another impression I have is that most of us have far more confidence in retirement models than our understanding of them merits, and "us" includes financial planners. I appreciate models as research and learning tools and as tools that can support a good retirement plan, but I've been building and studying them for perhaps 20 years and the list of their weaknesses is long. They can't predict an individual household's future and they are not by themselves a retirement plan (see Michael Kitces' recent post.)
Positive feedback
loops are a characteristic of chaos theory. Is our retirement income system a
chaotic system that is normally in equilibrium but can unpredictably be
drawn into the influence of a point attractor like ruin? I'm not
certain, but I have been doing some research and having some interesting
discussions. Check out Retirement Income and Chaos Theory.
Tuesday, December 1, 2015
100% Certain That We’re Not Sure
In my previous post, Uncertainty Denial, I promised to suggest a way to accept and plan for the uncertainty of future market returns rather than to deny it. Future market returns, future expenses and how long a healthy person will live are critical to your retirement plan and all are uncertain. The uncertainty is inescapable. Relying solely on averages or likelihoods in a retirement plan is risky.
In this post, I’ll talk about the uncertainty of market returns and the impact that has on our ability to optimize the financial parameters of a retirement plan, like spending rates, equity allocations and how much we need to fund a safe floor of income.
Let’s say you decide to base your plan on the assumption that your portfolio will return 5% annually in real dollars with a standard deviation of 11% over the remainder of your lifetime. You should have very little confidence that this prediction is in any way accurate, but let’s stick a pin in that for now and pretend that we’re pretty sure of our prediction.
That 11% standard deviation quantifies the uncertainty of our estimate. It means that roughly two-thirds of the annual returns will fall between a 6% loss and a 16% gain, a pretty broad range. About 95% of those returns will fall between a 17% loss and a 27% gain.
The following chart shows terminal portfolio values (wealth at death) from a Monte Carlo simulation for a retiree spending $40,000 a year from a $1M initial portfolio balance (the 4% rule) and living exactly 30 years in retirement. The red dots are scenarios that ended in ruin before 30 years. The lighter blue dots represent scenarios that survived 30 years but ended up with less than the $1M the scenarios began with. The dark blue dots represent scenarios that funded thirty years and ended up with more than the portfolio’s initial value. The probability of ruin is 6.7% for all scenarios.
(I fudged a bit on the TPV of the red dots by continuing spending after the portfolio was depleted for the sake of visual clarity. The values of these failed portfolios should all be precisely zero, but that places all the red dots on the x-axis and it is impossible to see how many there are.)
Figure 1 above shows a lot of uncertainty. About 14% of the scenarios had annual returns less than 2.5%, or half the expected return. About 11% had returns greater than 7.5%, or 50% greater than the expected 5%. Figure 2 below shows the same simulation as above, but adds life expectancy modeled from mortality tables for a 65-year old couple.
There are several points of interest regarding Figure 2. First, the points are more scattered than the first graph, showing the degree of uncertainty that life expectancy adds. That means real retirement outcomes are less predictable than studies assuming a 30-year retirement. Second, there are fewer red dots in this chart than in Figure 1 because many retirees who would have run out of money had they lived 30 years would die before going broke in real life. (Only about 6% of 65-year old males will live another 30 years or more.)
The probability of ruin drops from 6.7% to 3% for all scenarios when we include life expectancy in the model. This is consistent with a study by Stout and Mitchell that found that considering life expectancy in the sustainable withdrawals rate (SWR) model lowers the probability of ruin by about half. That’s another way of saying that fixed-length retirement models overstate the probability of ruin.
While 3% of the 10,000 scenarios ended with failed portfolios before death, 82% of those failures (1,400/1,700) occurred when returns were worst, 18% occurred with below average returns, and 0.01% occurred when returns were greater than the expected 5%.
Shorter lifetimes than 30 years means less time to grow those big terminal portfolios found in the upper right quadrant of both figures, and when joint lifetimes for a couple are considered instead of assuming they always retire for 30 years, the median terminal portfolio value falls 22% in this example.
Those huge terminal portfolio values from SWR studies that leave us starry-eyed are possible, but they require all four of these pieces of good luck: excellent market returns, a long life, a fortunate sequence of those returns, and no spending shocks. On the other hand, any one of poor market returns, an unfortunate sequence of returns, or spending shocks combined with a long life can be financially catastrophic.
Third, notice the dots (scenario outcomes) in Figure 2 near the 5% annual return line. The outcomes range from a couple of failed scenarios (red dots) to one leaving about $3M to heirs. Outcomes range from portfolio failure to a huge success even though they all experienced a 5% average annual return on investments. Or look at the outcomes with an annual average 10% return that range from $1M to $8M. These examples illustrate sequence of returns risk.
Next, notice that there is one lonely failed scenario in the Above Average Returns range and none in the Best Returns range. Had I increased the number of simulations from say, 10,000 to 100,000, more failures would show up in all ranges and a few would appear in the Best Returns range. These additional failures are improbable, but not impossible. The best range of returns is not immune to portfolio failure; failures there are just less likely.
Lastly – and perhaps most importantly, if you retired 10,000 times then your history of terminal portfolio balances might look a lot like Figure 2. But unless you're Dr. Who, you only get one retirement and one of those dots.
(If you are Dr. Who, please call me. I need to know if Clara is permanently dead. The suspense is killing me.)
Table 1 below shows the statistics for the simulation that includes joint life expectancy.
Next, let’s add rows to Table 2 for the 95th-percentile sustainable spending rate using Milevsky’s formula and the recommended asset allocation using the AACalc website. Those last two rows of Table 2 show the spending rate and asset allocation we would have chosen had we known we would end up with those returns instead of the 5% average return that we assumed.
And herein lies the planning predicament. Although 5% returns with an 11% standard deviation sounded precise, it actually provides a 1-in-7 possibility of a terrible outcome and nearly a 1-in-5 possibility of a really great outcome. For which scenario do we plan? Worst Returns with 1.1% annual spending and a 15% equity allocation? Best Returns with a 5.9% spending rate and 95% equity allocation? Somewhere in the middle?
A lot of plans will focus on the middle, but a good plan will consider them all.
Here’s my thinking. The first order of business is to eliminate catastrophes – those red dots. We can’t avoid red dots when we fund retirement with an equity portfolio because even good returns on average can fall victim to an unfortunate sequence of returns. We can, however, make red dots non-catastrophic by building a floor of safe income with TIPS bond ladders, Social Security benefits, fixed annuities and the like to insure that we can meet our non-discretionary spending needs. Then, even if our equity portfolio becomes a red dot, we don’t lose our standard of living.
Do this step first because most American households will find that once they allocate their assets to the floor and set aside some cash for liquidity, there won’t be much left to invest for upside. Floors are expensive, especially in the current low-interest rate environment. Most households won’t even be able to build an adequate floor. For those who can, the cost of the floor is likely to significantly change the size of the upside equity portfolio.
If you end up in Above Average Returns or Best Returns, you won’t have many money problems. I would hope for those outcomes, but not plan on them. We should plan for somewhere between Worst Returns and Below Average Returns, but this is still a broad range of equity allocations and spending rates. By doing so, we reduce the upside potential of better returns, but that is the cost of safety.
Many retirement income plans appear to make assumptions about these parameters that are far more certain than we can actually calculate. If the right answer is somewhere between 15% and 55%, tweaking an allocation by 5% seems hard to defend.
Whether I would choose closer to the Worst Returns or to the Below Average Returns parameters would depend largely on my capacity to build a floor. With a larger floor, I can take more equity risk and lean toward Below Average Returns. I also consider short-term volatility in setting my equity allocation, as I explained in The Role of Xanax in Asset Allocations. That might overrule these recommended allocations.
Regardless, retirees should revisit this decision annually because some uncertainty is reduced with time. As we age, for example, our remaining lifetime becomes more certain and the range of possible TPV's becomes more clear.
Now, I have to return to my earlier statement above that these calculations are based on the assumption that we are pretty confident in our prediction of 5% future returns with an 11% standard deviation – and that we have no reason to be.
To quote a recent study by Pastor and Stambaugh entitled, “Are Stocks Really Less Volatile in the Long Run?” (they aren’t, by the way):
The process I suggest may seem pointless given the amount of uncertainty involved, but a plan that results from our best efforts to quantify that uncertainty is better than no plan at all. If we can’t identify a plan that will work with certainty, we can at least rule out plans that probably won’t work and identify plans that are more likely to work. But, we have to recognize that uncertainty, even in the latter.
I suspect that most planners put way more faith in expected outcomes than is prudent. The unexpected outcomes are the ones to worry about.
The best strategy is to "buy insurance" to avoid catastrophic outcomes and invest what’s left. We buy portfolio failure insurance by building a floor of Social Security and pension benefits, TIPS bond ladders, and fixed annuities and we maximize that floor by delaying Social Security benefits as long as we can.
If we’re lucky, our wealth will grow. If we’re unlucky, we’ll still have a roof over our heads.
Don’t confuse precise probability calculations with certainty. As Jerry Horne said to his evil brother, Ben, after torching their lumber mill for insurance fraud in the second season of Twin Peaks, “Looks like we’re 100% certain that we’re not sure.”
Please check out my next post in this series, Positive Feedback Loops: The Other Roads to Ruin.
In this post, I’ll talk about the uncertainty of market returns and the impact that has on our ability to optimize the financial parameters of a retirement plan, like spending rates, equity allocations and how much we need to fund a safe floor of income.
Let’s say you decide to base your plan on the assumption that your portfolio will return 5% annually in real dollars with a standard deviation of 11% over the remainder of your lifetime. You should have very little confidence that this prediction is in any way accurate, but let’s stick a pin in that for now and pretend that we’re pretty sure of our prediction.
That 11% standard deviation quantifies the uncertainty of our estimate. It means that roughly two-thirds of the annual returns will fall between a 6% loss and a 16% gain, a pretty broad range. About 95% of those returns will fall between a 17% loss and a 27% gain.
The following chart shows terminal portfolio values (wealth at death) from a Monte Carlo simulation for a retiree spending $40,000 a year from a $1M initial portfolio balance (the 4% rule) and living exactly 30 years in retirement. The red dots are scenarios that ended in ruin before 30 years. The lighter blue dots represent scenarios that survived 30 years but ended up with less than the $1M the scenarios began with. The dark blue dots represent scenarios that funded thirty years and ended up with more than the portfolio’s initial value. The probability of ruin is 6.7% for all scenarios.
(I fudged a bit on the TPV of the red dots by continuing spending after the portfolio was depleted for the sake of visual clarity. The values of these failed portfolios should all be precisely zero, but that places all the red dots on the x-axis and it is impossible to see how many there are.)
Figure 1 above shows a lot of uncertainty. About 14% of the scenarios had annual returns less than 2.5%, or half the expected return. About 11% had returns greater than 7.5%, or 50% greater than the expected 5%. Figure 2 below shows the same simulation as above, but adds life expectancy modeled from mortality tables for a 65-year old couple.
There are several points of interest regarding Figure 2. First, the points are more scattered than the first graph, showing the degree of uncertainty that life expectancy adds. That means real retirement outcomes are less predictable than studies assuming a 30-year retirement. Second, there are fewer red dots in this chart than in Figure 1 because many retirees who would have run out of money had they lived 30 years would die before going broke in real life. (Only about 6% of 65-year old males will live another 30 years or more.)
The probability of ruin drops from 6.7% to 3% for all scenarios when we include life expectancy in the model. This is consistent with a study by Stout and Mitchell that found that considering life expectancy in the sustainable withdrawals rate (SWR) model lowers the probability of ruin by about half. That’s another way of saying that fixed-length retirement models overstate the probability of ruin.
While 3% of the 10,000 scenarios ended with failed portfolios before death, 82% of those failures (1,400/1,700) occurred when returns were worst, 18% occurred with below average returns, and 0.01% occurred when returns were greater than the expected 5%.
Shorter lifetimes than 30 years means less time to grow those big terminal portfolios found in the upper right quadrant of both figures, and when joint lifetimes for a couple are considered instead of assuming they always retire for 30 years, the median terminal portfolio value falls 22% in this example.
Those huge terminal portfolio values from SWR studies that leave us starry-eyed are possible, but they require all four of these pieces of good luck: excellent market returns, a long life, a fortunate sequence of those returns, and no spending shocks. On the other hand, any one of poor market returns, an unfortunate sequence of returns, or spending shocks combined with a long life can be financially catastrophic.
Third, notice the dots (scenario outcomes) in Figure 2 near the 5% annual return line. The outcomes range from a couple of failed scenarios (red dots) to one leaving about $3M to heirs. Outcomes range from portfolio failure to a huge success even though they all experienced a 5% average annual return on investments. Or look at the outcomes with an annual average 10% return that range from $1M to $8M. These examples illustrate sequence of returns risk.
Next, notice that there is one lonely failed scenario in the Above Average Returns range and none in the Best Returns range. Had I increased the number of simulations from say, 10,000 to 100,000, more failures would show up in all ranges and a few would appear in the Best Returns range. These additional failures are improbable, but not impossible. The best range of returns is not immune to portfolio failure; failures there are just less likely.
Lastly – and perhaps most importantly, if you retired 10,000 times then your history of terminal portfolio balances might look a lot like Figure 2. But unless you're Dr. Who, you only get one retirement and one of those dots.
(If you are Dr. Who, please call me. I need to know if Clara is permanently dead. The suspense is killing me.)
Table 1 below shows the statistics for the simulation that includes joint life expectancy.
Table 1. Portfolio Return Scenarios Including Joint Life Expectancy | ||||
---|---|---|---|---|
Outcome | Worst Returns | Below Average Returns | Above Average Returns | Best Returns |
Range of Returns | <= 2.5% | 2.5% to 5% | 5% to 7.5% | > 7.5% |
% of Scenarios | 14% | 33% | 34% | 18% |
Median Successful TPV ($) | 441,160 | 905,200 | 1,883,960 | 3,314,862 |
% Failed Scenarios | 14% | 3% | 0% | 0% |
Next, let’s add rows to Table 2 for the 95th-percentile sustainable spending rate using Milevsky’s formula and the recommended asset allocation using the AACalc website. Those last two rows of Table 2 show the spending rate and asset allocation we would have chosen had we known we would end up with those returns instead of the 5% average return that we assumed.
Table 2. SWR and Equity Allocation Recommendations | ||||
---|---|---|---|---|
Outcome | Worst Returns | Below Average Returns | Above Average Returns | Best Returns |
Range of Returns | <= 2.5% | 2.5% to 5% | 5% to 7.5% | > 7.5% |
% of Scenarios | 14% | 33% | 34% | 18% |
Mean Annual Return | 1.0% | 3.9% | 6.1% | 9.3% |
% Failed Scenarios | 14% | 3% | 0% | 0% |
Sustainable Spending Rate | 1.1% | 2.6% | 3.9% | 5.9% |
Equity Allocation | 15% | 55% | 100% | 95% |
And herein lies the planning predicament. Although 5% returns with an 11% standard deviation sounded precise, it actually provides a 1-in-7 possibility of a terrible outcome and nearly a 1-in-5 possibility of a really great outcome. For which scenario do we plan? Worst Returns with 1.1% annual spending and a 15% equity allocation? Best Returns with a 5.9% spending rate and 95% equity allocation? Somewhere in the middle?
A lot of plans will focus on the middle, but a good plan will consider them all.
[Tweet this]"Looks like we’re 100% certain that we’re not sure." — Jerry Horn, Twin Peaks.
Here’s my thinking. The first order of business is to eliminate catastrophes – those red dots. We can’t avoid red dots when we fund retirement with an equity portfolio because even good returns on average can fall victim to an unfortunate sequence of returns. We can, however, make red dots non-catastrophic by building a floor of safe income with TIPS bond ladders, Social Security benefits, fixed annuities and the like to insure that we can meet our non-discretionary spending needs. Then, even if our equity portfolio becomes a red dot, we don’t lose our standard of living.
Do this step first because most American households will find that once they allocate their assets to the floor and set aside some cash for liquidity, there won’t be much left to invest for upside. Floors are expensive, especially in the current low-interest rate environment. Most households won’t even be able to build an adequate floor. For those who can, the cost of the floor is likely to significantly change the size of the upside equity portfolio.
If you end up in Above Average Returns or Best Returns, you won’t have many money problems. I would hope for those outcomes, but not plan on them. We should plan for somewhere between Worst Returns and Below Average Returns, but this is still a broad range of equity allocations and spending rates. By doing so, we reduce the upside potential of better returns, but that is the cost of safety.
Many retirement income plans appear to make assumptions about these parameters that are far more certain than we can actually calculate. If the right answer is somewhere between 15% and 55%, tweaking an allocation by 5% seems hard to defend.
Whether I would choose closer to the Worst Returns or to the Below Average Returns parameters would depend largely on my capacity to build a floor. With a larger floor, I can take more equity risk and lean toward Below Average Returns. I also consider short-term volatility in setting my equity allocation, as I explained in The Role of Xanax in Asset Allocations. That might overrule these recommended allocations.
Regardless, retirees should revisit this decision annually because some uncertainty is reduced with time. As we age, for example, our remaining lifetime becomes more certain and the range of possible TPV's becomes more clear.
Now, I have to return to my earlier statement above that these calculations are based on the assumption that we are pretty confident in our prediction of 5% future returns with an 11% standard deviation – and that we have no reason to be.
To quote a recent study by Pastor and Stambaugh entitled, “Are Stocks Really Less Volatile in the Long Run?” (they aren’t, by the way):
“Expected return is notoriously hard to estimate. . . “estimation risk” . . . reflects the fact that, after observing the available data, an investor remains uncertain about the parameters of the joint process generating returns, expected returns, and the observed predictors. That parameter uncertainty adds to the overall variance of returns [risk] assessed by an investor.”In other words, we are adding to the uncertainty quantified by an 11% standard deviation for our 5% return assumption the uncertainty that 5% and 11% were the right guesses in the first place. My calculations above, for example, only show the uncertainty assuming we were certain about our return assumption. Were 4% a better estimate of future returns, for example, sustainable spending rates would have ranged from 0.7% to 5.2% and equity allocation recommendations from 0% to 95%, a sizable difference from the 5% guesstimate.
The process I suggest may seem pointless given the amount of uncertainty involved, but a plan that results from our best efforts to quantify that uncertainty is better than no plan at all. If we can’t identify a plan that will work with certainty, we can at least rule out plans that probably won’t work and identify plans that are more likely to work. But, we have to recognize that uncertainty, even in the latter.
I suspect that most planners put way more faith in expected outcomes than is prudent. The unexpected outcomes are the ones to worry about.
The best strategy is to "buy insurance" to avoid catastrophic outcomes and invest what’s left. We buy portfolio failure insurance by building a floor of Social Security and pension benefits, TIPS bond ladders, and fixed annuities and we maximize that floor by delaying Social Security benefits as long as we can.
If we’re lucky, our wealth will grow. If we’re unlucky, we’ll still have a roof over our heads.
Don’t confuse precise probability calculations with certainty. As Jerry Horne said to his evil brother, Ben, after torching their lumber mill for insurance fraud in the second season of Twin Peaks, “Looks like we’re 100% certain that we’re not sure.”
Please check out my next post in this series, Positive Feedback Loops: The Other Roads to Ruin.
Subscribe to:
Posts (Atom)